Understanding Machine Learning: An Introduction
- blockchaindevelope8
- Dec 12, 2024
- 5 min read
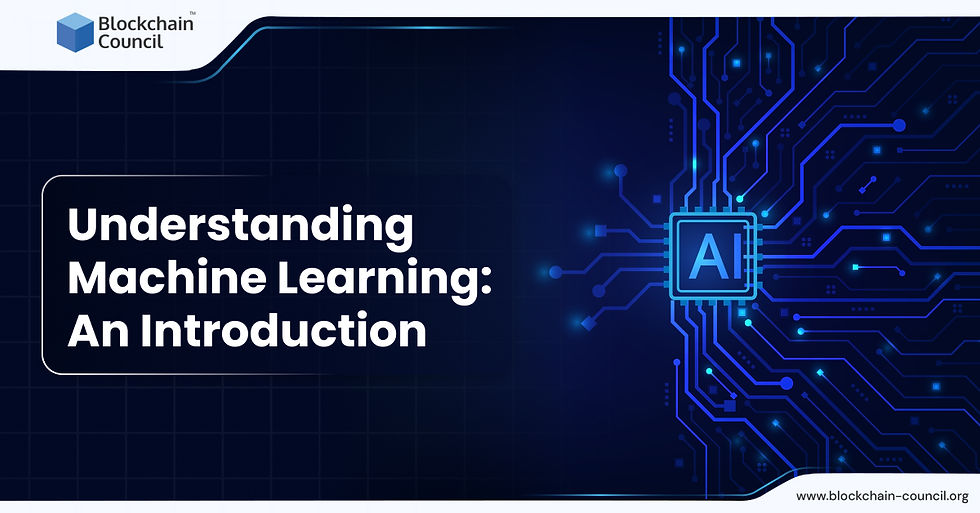
In the modern world, machine learning is a very potent and prominent technology that is growing in significance. We have yet to reach its full potential, which implies that its influence will only increase going forward. In the upcoming years, machine learning will continue to be a popular topic of conversation. By providing a thorough overview without delving too deeply into intricate details, this essay seeks to introduce the core ideas of machine learning.
Machine Learning: Revealing Data Transformation's Potential
One tool that transforms data into knowledge is machine learning. The world has seen unparalleled data growth during the last fifty years. However, once this data is not very valuable, we can examine it and identify significant trends. Intricate decision-making processes, future event prediction, and the automatic identification of sound patterns in large datasets are all made possible by machine learning approaches.
As noted futurist John Naisbitt eloquently stated, "We are starving for knowledge and drowning in information." By revealing hidden patterns and knowledge within enormous datasets, machine learning tackles this problem. It advances various industries, from self-driving cars and cancer diagnosis to music suggestions and Google searches.
The Development of Systems Other Than Rule-Based
Machine learning is especially intriguing since it deviates from conventional rule-based systems. Software engineering is used to tackle issues by combining data and human-made rules. In contrast, machine learning uses data and solutions to identify the underlying rules governing an issue rather than explicit rules.
Machines explore various rules and learn from their performance to understand them. The core of machine learning is this iterative learning process, which differs from traditional methods where humans explicitly define rules.
Words and phrases Deconstructed
It's important to become familiar with several essential terms before delving into the complexities of machine learning:
Dataset: An assortment of data examples with characteristics necessary for solving problems.
Qualities: Important bits of information that help with problem comprehension are supplied into machine learning algorithms.
A model represents a phenomenon that a machine learning algorithm has learned from training data. It functions as the result of training.
The Unveiling of the Machine Learning Process
To comprehend machine learning, one must realize its sequential steps:
Data collection: Compiling information to learn algorithms.
Data preparation includes lowering dimensionality, extracting essential features, and formatting and engineering data for best use.
Training: The algorithm learns from the gathered and prepared data during the fitting stage.
Evaluation: Putting the model to the test to see how well it works.
Tuning: Adjusting the model to run as efficiently as possible.
Following the Roots: Ada Lovelace and the Theory of Probability
Ada Lovelace, a computing pioneer who saw that machines might comprehend the world without human help, is credited with laying the groundwork for machine learning. About 200 years later, her idea aligns with the fundamental concepts of machine learning, which is the process of deriving mathematical correlations and patterns from data.
Thomas Bayes, a mathematician, developed probability theory essential to machine learning. Using a Bayesian perspective, machine learning sees probability as a way to measure an event's uncertainty based on current information. This method improves decision-making based on pertinent traits and reasoning by enabling the assigning of probabilities to uncommon events.
Various Methods for Machine Learning
A variety of methods, each suited to a particular situation, are included in machine learning. Among the main categories are:
Learning Under Supervision Unsupervised Learning
Reinforcement Learning in Semi-supervised Learning
Supervised Education: Connecting Inputs to Outcomes
Learning the mapping (rules) between inputs and outputs is the aim of supervised learning. The model is guided during learning by labeled data, such as previous input and output pairings. This method works well for jobs like making predictions based on past data.
Unsupervised Learning: Identifying Trends Without Classification
On the other hand, unsupervised learning uses input data and produces unlabeled outputs. Unsupervised learning—which includes methods like clustering, association, anomaly detection, and dimensionality reduction—is excellent at revealing complex patterns in data, even in the absence of labeled instances.
Semi-supervised Education: Finding Equilibrium
A compromise between supervised and unsupervised methods is achieved by semi-supervised learning. This method expands the range of issues machine learning can handle by fusing a small piece of labeled data with a larger unlabeled dataset. One noteworthy development in semi-supervised learning is the appearance of Generative Adversarial Networks (GANs), which have shown impressive outcomes when using sparsely labeled data.
Reinforcement Learning: Acquiring Knowledge Through Incentives
Reinforcement learning mimics human learning by depending on rewards rather than clear labels. Like teaching a dog with goodies, positive and negative feedback can either encourage or discourage certain behaviors. Despite its complexity, this method allows machines to learn in dynamic, data-rich contexts by breaking away from static datasets.
Applications in the Real World: Filling the Theory-Practice Gap
Although machine learning's theoretical underpinnings are fascinating, its transformational potential is demonstrated by practical implementations. The applications are numerous and significant, from finding new star types through clustering in unsupervised learning to lowering data center expenses through efficient cooling system control utilizing reinforcement learning.
What is Certification in AI? Increasing Knowledge in the Changing AI Environment
Getting AI certifications is becoming increasingly important to keep ahead of technical advancements and improve professional competence as artificial intelligence (AI) and machine learning take the globe by storm. AI certification addresses different aspects of AI applications. Examining different AI expert certificates becomes essential for anyone hoping to learn more about the complexities of AI technologies.
As a result, an AI certification test guarantees a uniform gauge of competency by attesting to a person's skill and comprehension of AI principles. As the need for certified chatbot specialists and AI developers grows, these credentials will confirm a person's skill set and provide access to profitable possibilities across a range of industries. In addition to improving one's ability to create complex AI algorithms, mastery of AI technologies makes it possible to integrate AI chatbots for more efficient and intelligent interactions throughout the ecosystem. Thus, taking the top AI developer certification courses has become essential for professionals trying to understand the intricacies of the AI field, encouraging creativity and guaranteeing a competitive advantage in today's fast-paced, rapidly changing digital world.
In summary: Managing Machine Learning's Future
We are on the verge of a technological revolution when we peel back the layers of machine learning, from its historical foundations to its various methodologies and practical applications. Knowing machine learning is essential for navigating the future when data-driven insights and clever algorithms shape our world, and it goes beyond simple academic study. Machine learning is just starting in this age of rapid technological improvement, yet it promises ongoing innovation and previously unheard-of breakthroughs.
The Blockchain Council is a source of knowledge for anyone wishing to start a path of ongoing education and certification in cutting-edge technologies. The Blockchain Council, committed to promoting blockchain research and development, use cases, and solutions for a better world, is led by subject matter experts and enthusiasts.
As evidence of its dedication to remaining on the cutting edge of technology, the Blockchain Council provides a range of AI prompt engineer credentials and chatbot certification courses. For professionals looking to advance their careers and contribute to the rapidly changing fields of artificial intelligence and machine learning, the Blockchain Council is an invaluable resource.
Comentarios